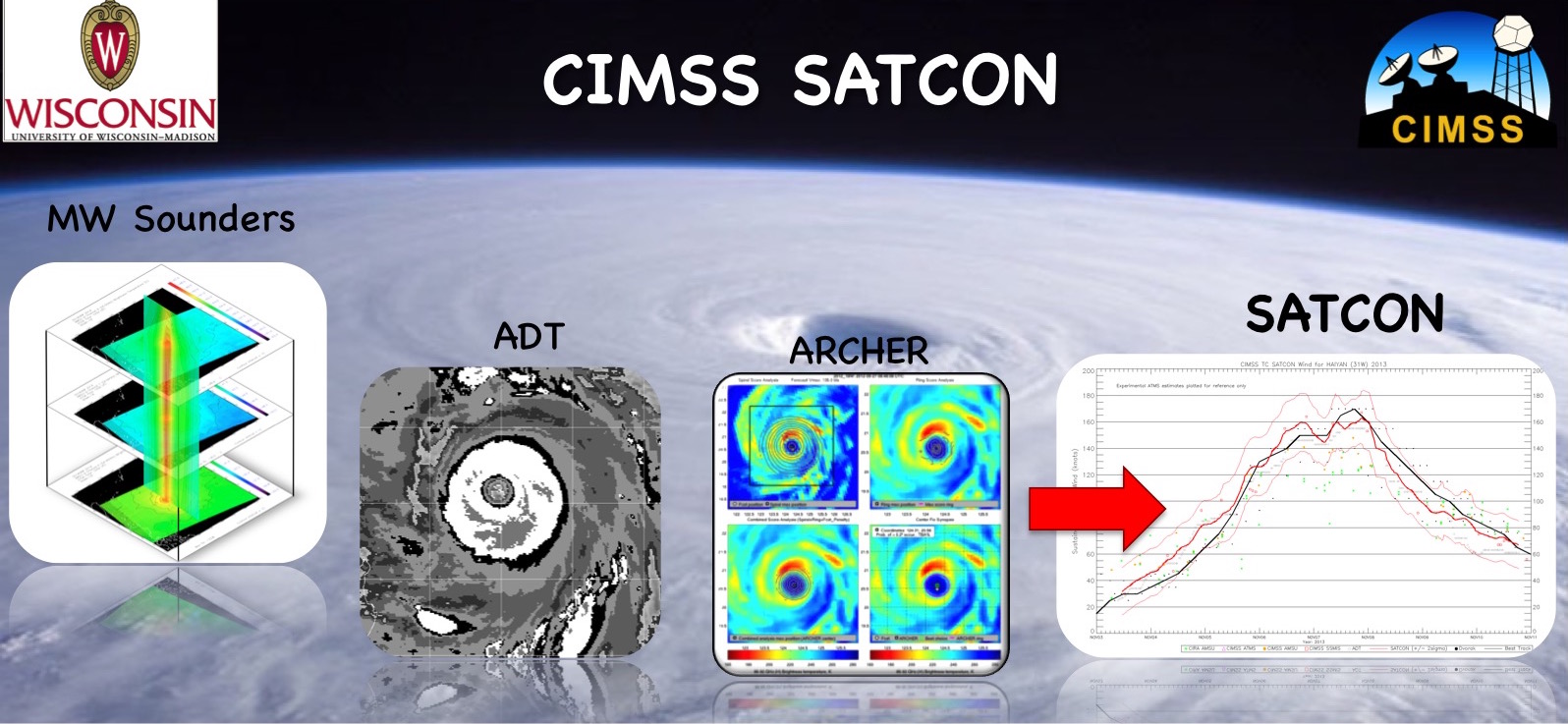
The CIMSS Satellite Consensus (SATCON) product blends tropical cyclone intensity estimates derived from multiple objective algorithms to produce an ensemble estimate of intensity for current tropical cyclones worldwide. The algorithm uses individual ADT, CIMSS AMSU, CIMSS SSMIS, CIMSS and CIRA ATMS intensity estimates utilizing a statistically-derived weighting scheme which maximizes/minimizes the strength/weaknesses of each technique to produce a consensus estimate of the current tropical cyclone intensity. The goal of this work is to produce an estimate of intensity that is superior to the individual components. Statistical verification of the method has shown it to be comparable in skill to the Dvorak Technique used by tropical cyclone warnings agencies. In some situations the algorithm can outfperform the Dvorak Technique.
A statistical analysis of each of the member algorithms was used to determine the individual member performance in a variety of TC structures. Each algorithm has strengths and weaknesses that are a function of the algorithm limitations, scanning geometry, instrument resolution or a combination of these factors. For example the ADT algorithm assigns a scene type to each IR image of a TC. ADT performance is strongly dependent on scene type with the best performance for scenes when a clear eye is present and decreased algorithm performance for other scenes. Because of this dependence the ADT is weighted according to scene type.
 |
Examples of ADT Scene Types
The microwave sounder algorithms are sensitive to TC core size as compared to the instrument resolution. Eye size information is provided by the CIMSS ARCHER algorithm, the ADT or the warning agency working Best Track in that order of availability. If the TC eye size is small than the sounder FOV resolution a correction is applied. In addition because of the relatively coarse instrument resolution it is possible that the true TC center may not be co-located with the instrument Field of View (FOV) used for the estimate. This source of under-sampling is addressed in AMSU using information from the AMSU-B moisture sounder. Microwave sounder performance is best when the TC eye is sufficiently large compared to the FOV resolution therefore the sounders are weighted according to whether or not the TC inner core is resolved. In the case of the CIRA ATMS algorithm only estimates where the TC eye size is larger than 40 km are used.
Because the ADT estimates are available for every infrared satellite image it is desireable to have matching microwave sounder estimates. To accomplish this the polar orbiting satellite estimates which are available only at irregular times are interpolated to hourly estimates. These interpolated estimates are then combined with the ADT estimates using the weighting approach. CIMSS ATMS estimates are treated as an SSMIS member because the two instruments have similar resolution/performance and this improves the fidelity of the interpolated values. SSMIS and ATMS sounder estimate weights are adjusted based on the age of the estimate using an exponential decay rate. Thus estimates older than 3 hours have decreasing weight which approaches zero once the estimate is older than 6 hours. This is done because there are currently only two sounders available at this resolution.
In order to account for storm structure differences that relate to TC intensity a Pressure-Wind (P-W) relationship-based SATCON estimate is produced. The P-W SATCON Vmax estimate uses the pressure anomaly derived from the SATCON estimate of MSLP and an estimate of the environmental pressure obtained from the warning agency (NHC or JTWC). This estimate is then corrected to account for bias as a function of latitude, storm size (distance to outer closed isobar also obtained from the warning agency), TC eye size (from ARCHER or ADT only), and intensity. The intensity bias correciton primarily adjusts the SATCON Vmax for a known slight too weak bias for the strongest storms. The final SATCON Vmax estimate is 75% of the SATCON Vmax members estimate and 25% of the SATCON P-W estimate.
The goal of SATCON is to produce an estimate of TC intensity that is superior to both the individual members and a simple average of the members. The tables below show the statistical performance of the algorithms for the 2006-2015 period. Validation consists of warning agency best track estimates of Vmax and MSLP during periods when aircraft ground truth was available within three hours of the intensisty estimate.
Poster from 2014 AMS Hurricane Conference Explaining the latest version of SATCON and Stats (PDF)
Go to SatCon Real-Time analysis page.